Introduction
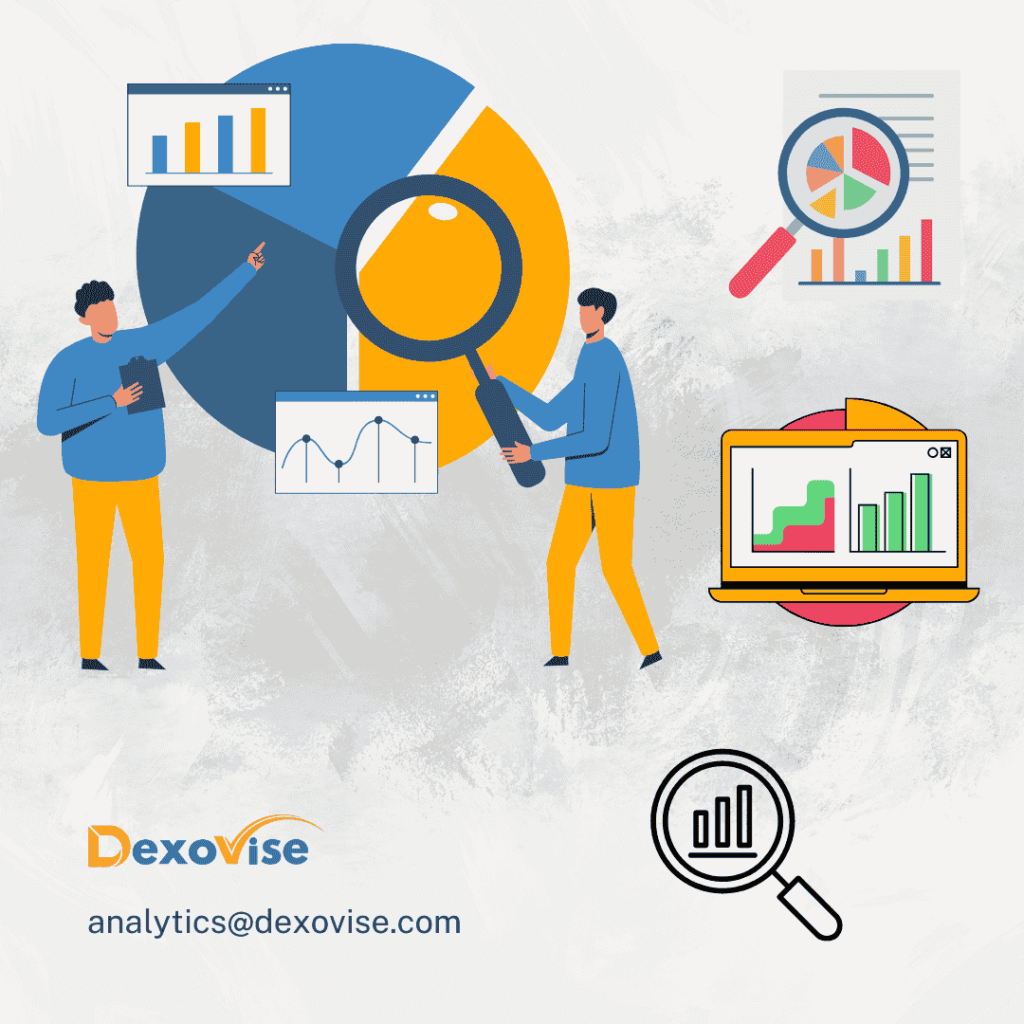
Data Analytics Trends 2025- why it’s important for your business? Picture this: It’s 2025, and the world of data analytics has undergone a seismic shift. 🚀 The tools and techniques we once relied on seem almost primitive compared to the cutting-edge technologies now at our fingertips. But how did we get here, and more importantly, where are we headed?
It’s not just an advantage anymore—it’s a necessity to remain on top of the race in this rapidly changing market. From AI-driven revolutions to the mind-bending possibilities of quantum computing, the future of data analytics promises to reshape industries, empower decision-makers, and unlock insights we once thought impossible. Do you want to know how data analysis will evolve in the future?
Join us as we explore seven game-changing trends that are set to transform the world of data analytics by 2025. We’ll uncover how edge computing is bringing analytics closer to the source, how augmented analytics is supercharging decision-making, and why ethical AI is no longer optional but essential. Get ready to glimpse the future and discover how to prepare for tomorrow’s data-driven world.
Struggling with Financial Clarity for Your Business?
Discover how our FP&A services help startups and SMBs optimize budgeting, improve forecasting, and make data-driven decisions for sustainable growth.
1. AI-Driven Analytics Revolution
Machine Learning-Powered Predictive Models
Machine learning revolutionizes predictive analytics, enabling businesses to make more accurate forecasts and decisions. By 2025, we expect to see:
Advanced algorithms capable of handling complex, multi-dimensional data
Real-time model updates for continuously evolving predictions
Integration with IoT devices for enhanced data collection and analysis
Here’s a comparison of traditional vs. ML-powered predictive models:
Feature | Traditional Models | ML-Powered Models |
---|---|---|
Data Handling | Limited to structured data | Can process structured and unstructured data |
Scalability | Limited | Highly scalable |
Accuracy | Moderate | High and improving over time |
Speed | Slower processing | Rapid analysis and predictions |
Adaptability | Requires manual updates | Self-learning and adaptive |
Natural Language Processing for Data Interpretation
Natural Language Processing (NLP) makes data analytics more accessible and intuitive. Key advancements include:
Conversational analytics interfaces
Automated report generation in natural language
Sentiment analysis for customer feedback and social media data
Automated Insight Generation
AI-driven analytics is moving beyond data processing to automated insight generation. This involves:
Identifying patterns and anomalies without human intervention
Suggesting actionable recommendations based on data analysis
Prioritizing insights based on business impact and relevance
These advancements in AI-driven analytics are setting the stage for more sophisticated and accessible data analysis tools. As we move forward, we’ll explore how edge computing brings these capabilities closer to the data source, enhancing real-time decision-making capabilities.
The Rise of Explainable AI
Explainable AI (XAI) is getting emerged as a crucial area of focus as organizations increasingly recognize the importance of transparency in AI systems. By elucidating the inner workings of AI operations, XAI significantly enhances the trustworthiness and reliability of these systems, fostering user confidence.
a) Transparency in Regulated Industries
This transparency is vital in highly regulated industries such as finance and healthcare, where ethical considerations and compliance with stringent regulatory norms are inevitable.
b) Clarifying Financial Decisions
Explainable algorithms play a crucial role in clarifying the processes behind loan approvals in financial decision-making. They ensure fairness by mitigating biases, addressing ethical concerns, supporting regulatory compliance, and building user trust.
c) Accelerating Adoption
As regulatory pressures continue to mount, the adoption of XAI is poised to accelerate. This trend underscores XAI’s pivotal role in advancing ethical and reliable AI practices, positioning it as a key development area for 2025 and beyond. The emphasis on explainability enhances the accountability of AI systems. It ensures they align with ethical standards and regulatory requirements, fostering greater trust and acceptance among users and stakeholders.
2. Edge Computing in Data Analytics
Edge computing is revolutionizing data analytics by enabling real-time processing at the source of data generation. This shift from centralized to decentralized processing offers numerous benefits:
Immediate insights
Reduced data transfer
Lower operational costs
Improved response times
For example, logistics companies use edge analytics to optimize delivery routes in real-time, cutting costs and improving service. The same technology reduces latency and enhances scalability in industries like healthcare, manufacturing, and smart cities.
By 2025, we expect to see a significant increase in edge devices capable of performing complex analytics tasks on-site.
Reduced Latency and Bandwidth Usage
One of the primary advantages of edge computing in data analytics is the dramatic reduction in latency and bandwidth consumption. Here’s a comparison of traditional vs. edge computing:
Aspect | Traditional Computing | Edge Computing |
---|---|---|
Latency | High | Very Low |
Bandwidth Usage | High | Low |
Data Transfer | Large volumes | Minimal |
Processing Location | Centralized data centers | Near data source |
This shift allows for near-instantaneous decision-making, crucial for applications like autonomous vehicles and industrial IoT.
Enhanced Data Privacy and Security
Edge computing significantly enhances data privacy and security in analytics:
Minimized data movement
Localized data processing
Reduced attack surface
Compliance with data regulations
By processing sensitive data locally, organizations can better adhere to strict data protection laws while still deriving valuable insights.
IoT Integration for Smarter Analytics
The integration of IoT devices with edge computing is set to transform data analytics by 2025. This combination enables:
Real-time monitoring and predictive maintenance
Smart city applications
Personalized customer experiences
Efficient supply chain management
As IoT deployments grow, edge analytics will become increasingly crucial for handling the massive influx of data generated by these devices.
3. Augmented Analytics for Enhanced Decision Making
AI-Assisted Data Preparation
AI-assisted data preparation is revolutionizing the way organizations handle their data. By automating complex tasks, it significantly reduces the time and effort required for data cleaning and preprocessing.
Automated data cleansing
Intelligent data integration
Smart feature engineering
Anomaly detection
Traditional Data Preparation | AI-Assisted Data Preparation |
---|---|
Time-consuming | Rapid and efficient |
Prone to human errors | Highly accurate |
Limited scalability | Easily scalable |
Requires extensive expertise | Accessible to non-experts |
Automated Data Discovery
Automated data discovery empowers users to uncover hidden patterns and insights without extensive manual exploration. This capability is transforming the way businesses derive value from their data assets.
Intuitive Visual Analytics Tools
Visual analytics tools are becoming increasingly intuitive, allowing users to interact with data in more natural and meaningful ways. These tools bridge the gap between complex data sets and human understanding, enabling faster and more informed decision-making.
Interactive dashboards
Natural language querying
Augmented reality data visualization
Collaborative data exploration
With these advancements, augmented analytics is set to become an indispensable tool for businesses seeking to gain a competitive edge through data-driven decision-making. As we move forward, we’ll explore how quantum computing is poised to revolutionize big data analytics, opening up new possibilities for processing and analyzing vast amounts of information.
4. Quantum Computing's Impact on Big Data
Solving Complex Analytical Problems
Quantum computing is poised to revolutionize data analytics by tackling complex problems that are currently unsolvable with classical computers. These machines leverage quantum mechanics principles to process information in ways that exponentially increase computational power.
Optimization problems
Financial modeling
Drug discovery simulations
Climate change predictions
Problem Type | Classical Computing | Quantum Computing |
---|---|---|
Optimization | Days or weeks | Minutes or hours |
Simulations | Limited accuracy | High precision |
Modeling | Simplified models | Complex, realistic models |
Faster Processing of Massive Datasets
The ability of quantum computers to process enormous datasets simultaneously will transform big data analytics. This quantum parallelism allows for:
Rapid pattern recognition in vast datasets
Real-time analysis of streaming data
Efficient processing of multi-dimensional data
Quantum Machine Learning Algorithms
Quantum machine learning (QML) combines quantum computing with machine learning techniques, offering significant advantages over classical algorithms:
Improved clustering and classification
Enhanced neural network training
More accurate predictive models
QML Advantage | Impact on Analytics |
---|---|
Speed | Faster model training and deployment |
Accuracy | More precise predictions and insights |
Complexity | Ability to handle intricate, multifaceted problems |
As quantum computing continues to evolve, its integration with big data analytics will unlock new possibilities for solving previously intractable problems and extracting deeper insights from massive datasets. This synergy between quantum computing and data analytics is set to redefine the boundaries of what’s possible in fields ranging from scientific research to business intelligence.
5. Ethical AI and Responsible Analytics
a) Bias Detection and Mitigation
As AI systems become more prevalent in data analytics, addressing bias has become a critical concern. Organizations are implementing sophisticated bias detection algorithms to identify and mitigate unfair outcomes. These tools analyze datasets and model outputs for potential biases related to gender, race, age, or other protected attributes.
Bias Type | Detection Method | Mitigation Strategy |
---|---|---|
Statistical | Distribution analysis | Data resampling |
Algorithmic | Model auditing | Algorithm adjustment |
Human | Expert review | Diverse team involvement |
b) Explainable AI Models
Transparency in AI decision-making is crucial for building trust and ensuring accountability. Explainable AI (XAI) techniques are being developed to make complex models more interpretable:
LIME (Local Interpretable Model-agnostic Explanations)
SHAP (SHapley Additive exPlanations)
Decision trees and rule-based systems
These methods provide insights into how AI models arrive at their conclusions, enabling stakeholders to understand and validate the reasoning behind analytical outcomes.
c) Privacy-Preserving Analytics Techniques
With increasing data privacy concerns, organizations are adopting techniques that allow for meaningful analytics while protecting individual privacy:
Differential Privacy: Adding controlled noise to data
Federated Learning: Distributed model training without central data storage
Homomorphic Encryption: Performing computations on encrypted data
d) Ethical Guidelines for Data Usage
As we move towards more responsible analytics, establishing and adhering to ethical guidelines is paramount. These guidelines typically cover:
Data collection and consent
Fair representation and inclusion
Transparency in data processing and model deployment
Accountability for AI-driven decisions
Organizations are forming ethics boards and implementing governance frameworks to ensure compliance with these guidelines, fostering a culture of responsible innovation in data analytics.
6. Data Democratization and Literacy
Self-Service Analytics Platforms
Self-service analytics platforms are revolutionizing data democratization by empowering non-technical users to access, analyze, and derive insights from data without relying on IT departments or data scientists. These platforms offer intuitive interfaces, drag-and-drop functionalities, and pre-built templates that enable users to create reports, visualizations, and dashboards with ease.
Key features of self-service analytics platforms:
User-friendly interfaces
Drag-and-drop report builders
Pre-built templates and visualizations
Data exploration tools
Natural language querying
Platform Type | Advantages | Challenges |
---|---|---|
Cloud-based | Scalability, accessibility | Data security concerns |
On-premises | Data control, compliance | Limited accessibility |
Hybrid | Flexibility, customization | Complex implementation |
Data Literacy Training Programs
As organizations increasingly recognize the importance of data-driven decision-making, data literacy training programs are becoming essential. These programs aim to equip employees across all levels with the skills needed to understand, interpret, and effectively use data in their daily work.
Common components of data literacy programs:
Basic statistical concepts
Data visualization techniques
Critical thinking and data interpretation
Ethical considerations in data analysis
Introduction to common analytics tools
Collaborative Analytics Environments
Collaborative analytics environments foster a culture of data-driven decision-making by enabling teams to work together on data projects, share insights, and leverage collective expertise. These platforms combine data analysis tools with collaboration features, creating a centralized space for data-related activities.
Now that we’ve explored data democratization and literacy, let’s examine how cloud-native analytics platforms are shaping the future of data analytics.
7. Cloud-Native Analytics Platforms
Scalable and Flexible Data Processing
Cloud-native analytics platforms are revolutionizing the way organizations handle data processing. These platforms offer unparalleled scalability and flexibility, allowing businesses to adapt quickly to changing data volumes and processing requirements.
Elastic scaling: Automatically adjust resources based on demand
Microservices architecture: Enable independent scaling of components
Containerization: Facilitate rapid deployment and resource optimization
Feature | Benefit |
---|---|
Auto-scaling | Cost-effective resource utilization |
Distributed processing | Faster data analysis and insights |
On-demand provisioning | Reduced infrastructure management |
Multi-Cloud and Hybrid Deployments
Organizations are increasingly adopting multi-cloud and hybrid strategies to leverage the strengths of different cloud providers and on-premises infrastructure.
Serverless Analytics Solutions
Serverless analytics is gaining traction, offering a pay-per-use model that eliminates the need for infrastructure management.
Enhanced Data Integration Capabilities
Cloud-native platforms are improving data integration capabilities, enabling seamless connection of diverse data sources and formats.
As we move towards 2025, cloud-native analytics platforms will continue to evolve, offering more advanced features and integration capabilities. These advancements will pave the way for more efficient and cost-effective data analysis, empowering organizations to extract valuable insights from their data with greater ease and speed.
Conclusion
As we look ahead to 2025, the data analytics landscape is set to undergo significant transformations. AI-driven analytics will revolutionize how we process and interpret data, while edge computing will bring analytics closer to the source, enabling real-time insights. Augmented analytics will empower decision-makers with more accessible and actionable information, and quantum computing promises to tackle complex data challenges at unprecedented speeds.
Ethical considerations and responsible AI practices will become increasingly crucial as these technologies evolve. Organizations must prioritize data literacy and democratization to harness analytics’s power across all levels fully. Cloud-native platforms will continue to play a pivotal role in scaling analytics capabilities. By staying informed and adaptable to these emerging trends, businesses and professionals can position themselves at the forefront of the data analytics revolution, driving innovation and making data-driven decisions with greater confidence and accuracy.